Network monitoring has always been a critical function for businesses of all sizes. It ensures the smooth and efficient operation of IT infrastructure, enabling seamless communication, data flow, and application performance. However, traditional network monitoring methods are increasingly struggling to keep pace with the complexities of modern IT environments. The rise of cloud computing, virtualization, and the Internet of Things (IoT) has generated a massive explosion of data, making it difficult for human administrators to identify and address issues proactively.
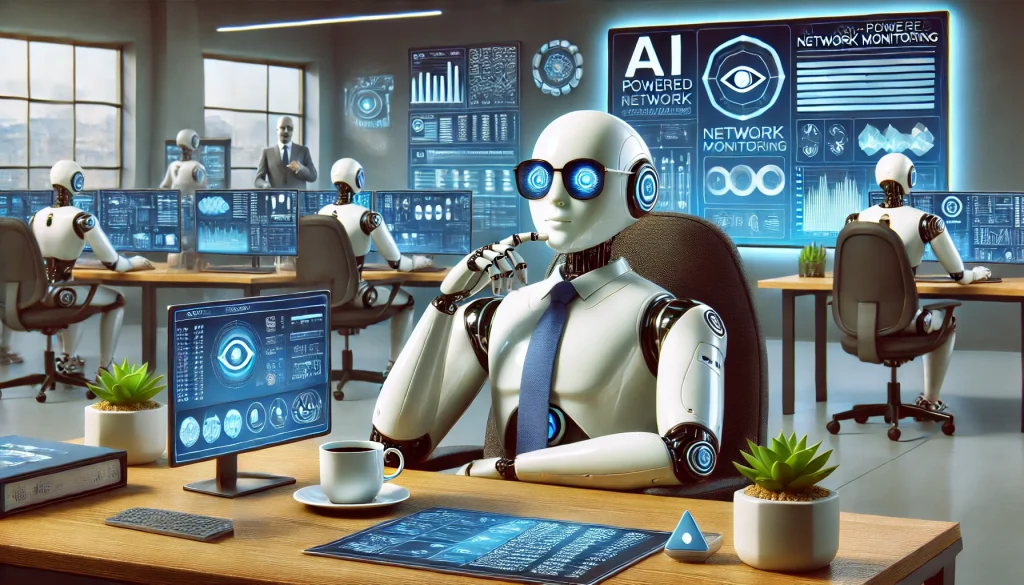
Enter Artificial Intelligence (AI) and Machine Learning (ML). These cutting-edge technologies are revolutionizing network monitoring, offering unprecedented levels of automation, intelligence, and efficiency. By leveraging the power of AI and ML, organizations can gain deeper insights into their network behavior, predict and prevent outages, and optimize performance with greater agility. And with the emergence of agentic AI, this transformation is poised to become even more profound.
1. The Challenges of Traditional Network Monitoring
Traditional network monitoring relies heavily on human intervention and rule-based systems. This approach presents several significant challenges:
- Data Overload: The sheer volume of data generated by modern networks can overwhelm human analysts, making it difficult to identify critical issues amidst the noise.
- Slow Response Times: Manual analysis and troubleshooting can be time-consuming, leading to slow response times to incidents and increased downtime.
- Limited Predictive Capabilities: Rule-based systems are often reactive, only alerting administrators after an issue has already occurred.
- Difficulty in Identifying Anomalies: Identifying unusual network behavior that may indicate a potential problem can be challenging, especially in complex and dynamic environments.
- Skill Shortages: Finding and retaining skilled network engineers with the expertise to manage and analyze complex networks is becoming increasingly difficult.
2. How AI and ML Are Transforming Network Monitoring
AI and ML are addressing these challenges by introducing several key capabilities:
- Automated Anomaly Detection: AI algorithms can analyze vast amounts of network data in real-time, identifying unusual patterns and deviations from normal behavior. This proactive approach allows organizations to address potential issues before they escalate into major outages.
- Predictive Maintenance: By analyzing historical data and identifying trends, ML models can predict potential future problems, such as equipment failures or bandwidth bottlenecks. This enables proactive maintenance and minimizes downtime. To illustrate, Cisco Catalyst™ Center, formerly Cisco DNA Center leverages AI/ML to automate network operations, providing insights and automating tasks.
- Root Cause Analysis: AI can quickly pinpoint the root cause of network issues, significantly reducing the time required for troubleshooting and resolution.
- Intelligent Alerting: AI-powered systems can prioritize alerts based on severity and potential impact, ensuring that critical issues are addressed first.
- Enhanced Security: ML algorithms can effectively detect and respond to cyber threats, such as DDoS attacks, malware infections, and insider threats.
3. Key AI and ML Techniques in Network Monitoring
Several AI and ML techniques are being applied to enhance network monitoring capabilities:
- Anomaly detection: Using AI to identify unusual network behaviors that could indicate potential problems like bottlenecks or failures by analyzing traffic patterns against established baselines.
- Predictive analytics: Analyzing historical network data to forecast future issues and proactively address them before they occur, utilizing machine learning models to identify patterns and trends.
- Network optimization: Real-time traffic analysis to optimize routing decisions, manage bandwidth allocation, and reduce latency by identifying congestion points. For instance, Juniper Networks’ Mist AI platform utilizes AI to optimize Wi-Fi performance, automate troubleshooting, and enhance user experience, demonstrating the potential for intelligent network management.
- Deep Learning: Deep learning algorithms, such as neural networks, can analyze complex data patterns and extract high-level features, enabling more accurate and insightful analysis.
- Security monitoring: Leveraging AI to detect malicious activity and potential security threats in network traffic, enabling faster response times to security incidents.
4. The Rise of Agentic AI in Network Monitoring
Agentic AI represents a significant leap forward in AI capabilities. Unlike traditional AI systems that primarily analyze data and provide insights, agentic AI systems can take actions, interact with their environment, and even collaborate with humans to achieve specific goals. In the context of network monitoring, this translates to:
- Self-Healing Networks: Agentic AI can automatically diagnose and remediate network issues without human intervention, minimizing downtime and ensuring continuous service availability.
- Automated Network Optimization: Agentic AI can continuously monitor and adjust network configurations to optimize performance, security, and resource utilization in real-time.
- Proactive Threat Response: Agentic AI can proactively identify and neutralize cyber threats before they can cause significant damage.
- Intelligent Collaboration: Agentic AI can collaborate with human network administrators, providing them with actionable insights and recommendations, and even automating complex tasks on their behalf.
5. The Importance of Enriched and Optimized NetFlow Data
Within the context of AI and ML-driven network monitoring, the quality of the input data – specifically NetFlow data – is paramount. Raw NetFlow data, while valuable, often lacks the depth and context necessary for AI/ML models to deliver their full potential.
- Enriched NetFlow: This involves augmenting basic NetFlow records with additional contextual information. This can include:
- Application Identification.
- User Identification.
- Geolocation.
- Device or VM Identification.
- Optimized NetFlow: This focuses on improving the efficiency and effectiveness of NetFlow data collection and processing. Key aspects include:
- Data Aggregation to reduce storage requirements and improve processing speed.
- Data Normalization by transforming data into a consistent format for easier analysis and comparison.
6. Real-World Applications of AI and ML in Network Monitoring
- Performance Monitoring and Optimization: AI and ML algorithms can analyze network performance metrics, such as latency, jitter, and packet loss, to identify bottlenecks and optimize network configurations for improved performance.
- Capacity Planning: AI-powered tools can forecast future network traffic demands, enabling organizations to proactively plan for capacity upgrades and avoid performance degradation.
- Security Threat Detection and Response: ML algorithms can effectively detect and respond to a wide range of cyber threats, including malware, DDoS attacks, and insider threats. For example, Splunk’s Security Operations Center (SOC) leverages AI and ML for threat hunting and response, automating security operations and demonstrating a step towards more autonomous security.
- Network Troubleshooting and Incident Management: AI can automate the process of identifying the root cause of network issues, significantly reducing the time required for troubleshooting and resolution.
- IT Operations Automation: AI and ML can automate many routine IT operations tasks, such as provisioning network devices, configuring firewalls, and managing network access.
7. The Benefits of AI and ML-Powered Network Monitoring
- Improved Network Visibility: Gain deeper insights into network behavior and identify potential issues before they impact business operations.
- Reduced Downtime: Minimize downtime by proactively identifying and addressing potential problems, and accelerating the resolution of incidents.
- Enhanced Security Posture: Effectively detect and respond to cyber threats, protecting critical business assets.
- Increased Efficiency and Productivity: Automate routine tasks, freeing up IT staff to focus on more strategic initiatives.
- Improved Cost-Effectiveness: Optimize resource utilization and reduce operational costs by identifying and eliminating inefficiencies.
8. Challenges and Considerations
- Data Quality: The accuracy and effectiveness of AI and ML models depend heavily on the quality of the data used for training.
- Data Privacy and Security: Organizations must ensure that sensitive network data is handled securely and in compliance with relevant regulations.
- Integration and Deployment: Integrating AI and ML solutions with existing network monitoring tools can be complex.
- Skill Development: Organizations need to invest in training and development programs to equip their IT teams with the necessary skills to effectively leverage AI and ML technologies.
- Explainability: Understanding how AI and ML models arrive at their conclusions is crucial for building trust and ensuring that decisions are made responsibly.
9. The Future of AI and ML in Network Monitoring
The future of network monitoring is bright, with AI and ML, particularly agentic AI, playing an increasingly critical role. As these technologies continue to evolve, we can expect to see even more sophisticated and intelligent solutions that can:
- Proactively predict and prevent future network issues with greater accuracy.
- Provide more personalized and context-aware network monitoring.
- Enable more autonomous and self-healing networks.
- Drive greater innovation in network design and architecture.
10. Conclusion
AI and ML are revolutionizing network monitoring, enabling organizations to gain unprecedented levels of visibility, control, and intelligence. By leveraging the power of these technologies, businesses can improve network performance, enhance security, and optimize IT operations for greater efficiency and agility. With the rise of agentic AI, we can expect even greater levels of automation, intelligence, and autonomy in network management. While challenges remain, the potential benefits of AI and ML-powered network monitoring are significant, and organizations that embrace these technologies will be well-positioned to thrive in the digital age.
By investing in the enrichment and optimization of NetFlow data, organizations can significantly enhance the value of their network monitoring efforts and unlock the full potential of AI and ML in this critical area.
Embracing AI and ML unlocks the full potential of networks, driving innovation, improving business agility, and gaining a competitive edge in today’s dynamic digital landscape.